
Machine Learning and Last-Mile Deliveries
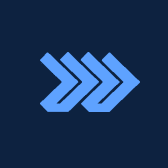
Wise Systems
Machine Learning and Last-Mile Deliveries
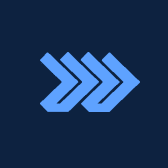
Wise Systems

Most of us would agree that experience matters. Whether you’re hiring a plumber, a pediatrician, a mechanic or a scientist, you typically choose the seasoned professional when given a choice. Why? Because experience generally has a strong correlation to positive future outcomes. Now, with artificial intelligence (AI) and machine learning (ML), machines (software) can now be ‘trained,’ which has profound implications for multiple industries, including last-mile deliveries.
Where humans learn from performing the same tasks time and again and absorbing the lessons from different circumstances, variables and outcomes, computers do the same. But rather than learning with their hands, computers and software learn from data and patterns found in data sets. With that experience, software can make better choices in the future to improve subsequent outcomes.
In last-mile operations, there are numerous elements, each with various complexities. There are depots, inventory, drivers and vehicles to consider, as well as customers, routes and — in the center of the system — dispatchers. All of these elements need to be perfectly choreographed to successfully execute a day’s deliveries on-time and on-plan. But those plans are often based on flawed assumptions. Here’s an example — service times.
Accurate plans depend on having accurate data, which is often a huge issue for many fleets that aren’t using mobile and cloud-based systems. The three graphs here illustrate why. The first (fig. 1) shows the static (projected) service times for a variety of locations that will receive deliveries, the second (fig. 2) shows actual service times, and the third (fig. 3) shows machine-learned service times.


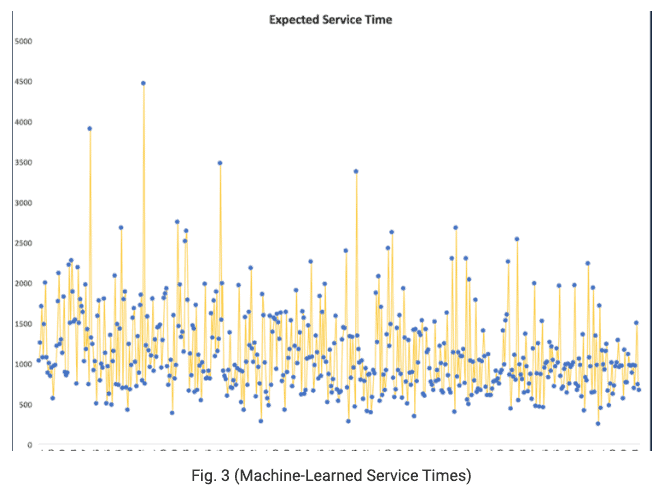
Now, consider the implications of building a route plan based on projected service times vs. machine-learned service times. In almost all instances, there’s variance — either minimal or significant — between expected and actual service times, and every one of those variations adds risk to a delivery plan. Through machine learning we can minimize that variance and the delta between planned and actual. The dynamic nature of the last mile means that there will never be a perfect plan, and service time is only one of the myriad issues drivers and dispatchers need to navigate in the course of a delivery day.
But with the availability of highly granular data about every aspect of the delivery day — from drivers’ preferred routes to learned service times and more — next-generation routing software has the opportunity to leverage machine learning to transform last-mile operations, and that’s what we do every day at Wise Systems.